Foundations for Meaning and Understanding in Human-centric AI
Carlo Santagiustina, VIU.
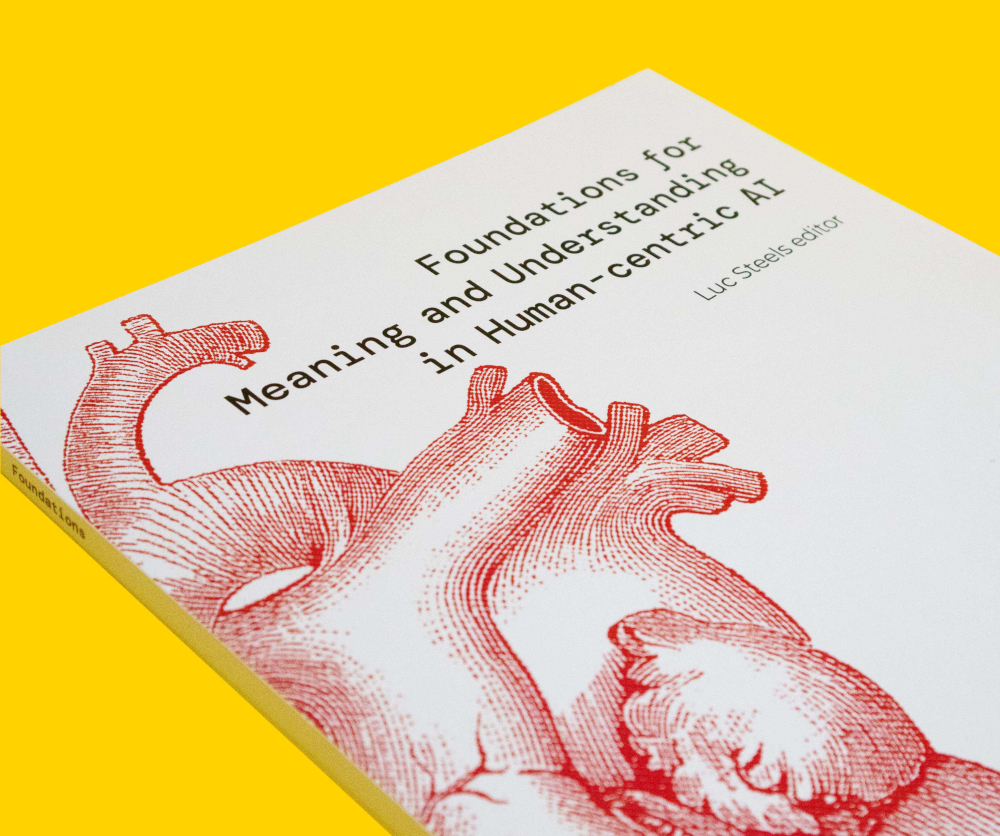
The summary that follows contains excerpts from the open-access volume of the MUHAI Deliverable 1.1:
Steels, Luc (ed.). (2022). Foundations for Meaning and Understanding in Human-centric AI. In Foundations for Meaning and Understanding in Human-centric AI (1-6-2022, p. 152) [Computer software]. Venice International University. https://doi.org/10.5281/zenodo.6666820
Through the Foundations for Meaning and Understanding in Human-centric AI, the MUHAI project offers an in-depth and integrated overview of narratives and understanding in different disciplines and research fields.
The volume builds upon recent insights and findings from social and cognitive sciences, humanities and other fields for which narratives have been found to play a relevant role in human understanding and decision-making processes. This, to map the state-of-the-art of narrative-centric studies and to identify the most promising research streams for tomorrow’s AI. In particular:
- Ch. 1 - Towards Meaningful Human-Centric AI, focuses on the conceptual foundations of human-centric AI and discusses some fundamental questions related to the proposed approach. Such as, what is the nature of meaning and understanding? And why is understanding needed to make AI more transparent and intelligible to humans?
- Ch. 2 - From Narrative Economics to Economists’ Narratives, explores the role of narratives in economic and social affairs, focusing on their usage for uncertainty avoidance, decision-making and decision justification. This chapter also highlights the strategic aspects of narratives and their relation to cognitive biases affecting humans’ sense- and decision-making processes.
- Ch. 3 - Narratives in Historical Sciences, highlights how Historical sciences try to offer causal explanations for non-recurrent phenomena, typically using incomplete and fragmentary evidence from the past. Through a case study on the French Revolution (1789–1799), this chapter shows in which terms narrative explanations go beyond mere description and have the potential for empirical testing.
- Ch. 4 - Clinical Narratives for Causal Understanding in Medicine, views clinical trials as causal narratives in the biomedical domain and as a means to understand the causal mechanisms of treatment effects. Through a use-case, this chapter describes what narratives in this domain are, and how they are formed and tested. This contribution also discusses how narratives can be represented computationally and how AI techniques can support domain experts in the generation of new hypotheses.
- Ch. 5 - Narratives in social neuroscience, suggests that narratives are a basic component of human cognition. Narratives are found to be a tool used by the human brain to make sense of experience and to build representations of the world and of ourselves. This chapter also presents studies about the area of moral values that show that the notion of narratives must take a central role in the future of social neuroscience and related fields.
- Ch. 6 - Narrative Art Interpretation, clarifies the narrative view on art interpretation with a concrete example of a painting by Caravaggio and it explores the implications of this view for building theories and mechanisms for dealing with meaning and understanding in AI systems.
- Ch. 7 - Pragmatics of Narration with Language, explores how research conducted into pragmatics and cognition can deepen our knowledge of natural languages, narratives, and ultimately language processing. The paper also illustrates how some of the fundamental pragmatic elements that characterise narratives heavily influence the ways languages structure basic linguistic elements, such as clauses, sentences, and texts.
As the conclusion of this volume highlights, narratives are ubiquitous and essential cognitive goods used in key spheres of our professional and social life, including the socio-economic, artistic, and scientific domain.
Through this work, the MUHAI consortium has undertaken a first (but far-reaching) step towards meaningful AI, proposing a variety of R&D paths to be explored, implemented, and tested in the next phases of the project. This kind of AI integrates and goes beyond ML and statistical methods for pattern recognition, completion and prediction, and explores how narrative-centric methods can inform the next generation of AI researchers and help them integrate in their systems narrative-related aspects of humans’ individual and collective understanding, which have yet to be fully acknowledged in AI research.
Our explorations have yielded a wealth of insights and possible applications of meaningful AI in a diverse set of fields, ranging from the analysis of debates about social inequality to hypothesis generation in scientific research.
By acknowledging the prominent role of narratives in understanding, the volume tries to facilitate the development of new methods that can better complement human understanding processes, eventually helping us identify and mitigate some cognitive biases that relate to narrative fallacies. Meaningful AI methods should be designed to fit to real world situations where inputs are typically sparse, fragmentary, ambiguous, underspecified, uncertain, vague, occasionally contradictory, and possibly deliberately biased, for example, because the producer of inputs is trying to deceive or manipulate. For understanding inputs with the aforementioned characteristics, different solution paths may have to be considered at the risk of exploding combinatorial complexity.
Understanding may be hard for AI systems because of the hermeneutic paradox: to understand the whole, you need to understand the parts, but to understand the parts, you need to understand the whole. This calls for integrated control (and meta control) structures other than a simple linear flow of processes organised in an automated pipeline.
As this volume suggests, meaningful AI models should be inspired by recent works in the field of knowledge representation, knowledge-based systems, fine-grained language processing and semantic web technologies, as well as other non-AI research streams explored in this volume, that we recommend you to read.
Foundations for Meaning and Understanding in Human-centric AI can be downloaded at this link: https://doi.org/10.5281/zenodo.6666820
More Articles
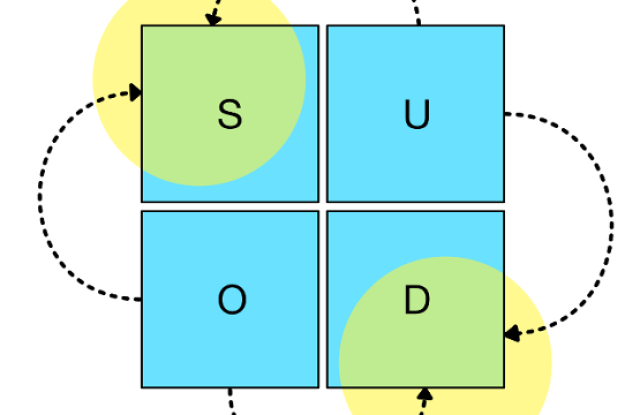
Uncommon Ground
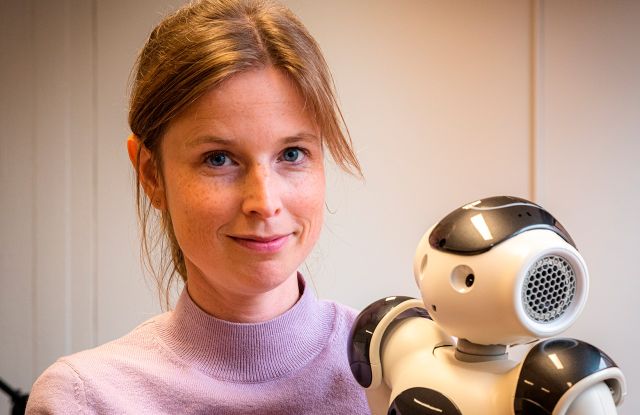
Do you speak AI?
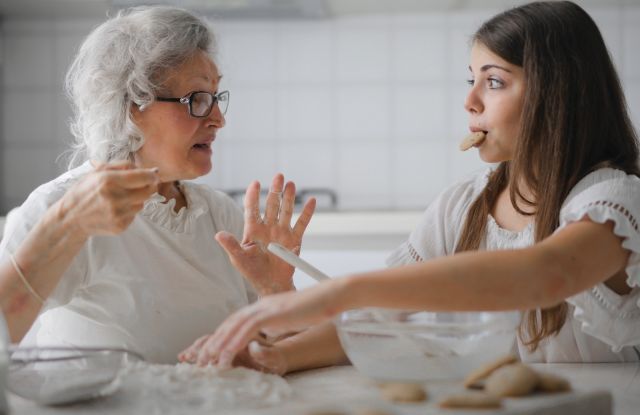
Pragmatics: the secret ingredient
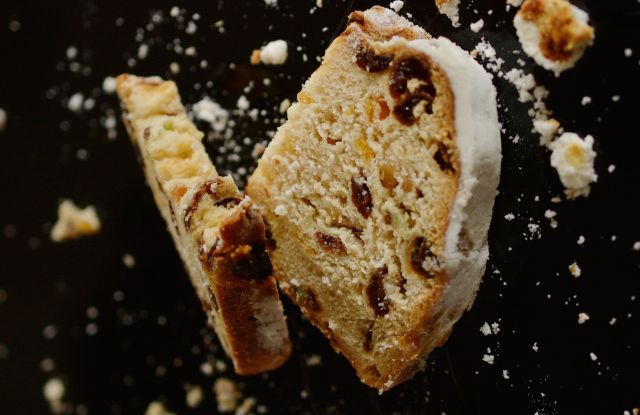
Deconstructing Recipes
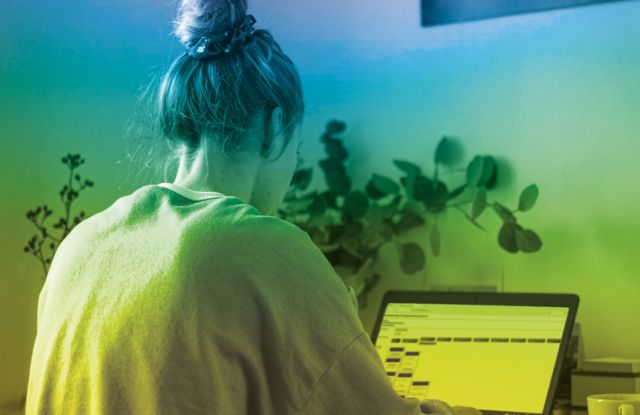
The FCG Editor: a new milestone for linguistics and human-centric AI
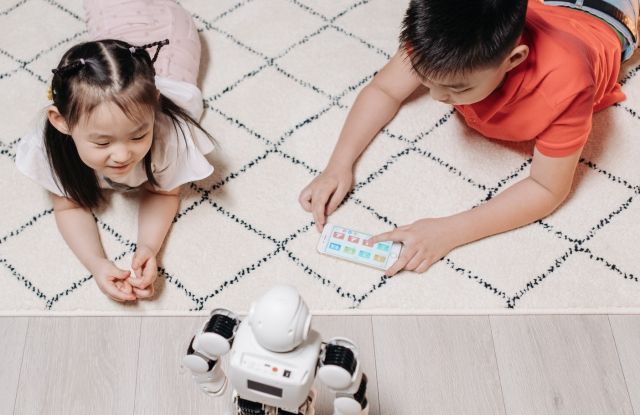
Linguistic Alignment for Chatbots
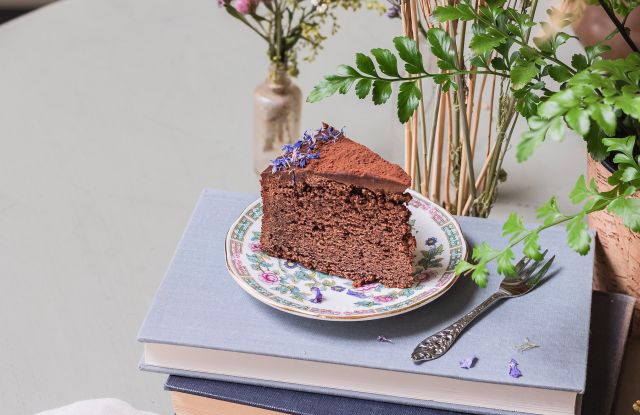
Framing reality
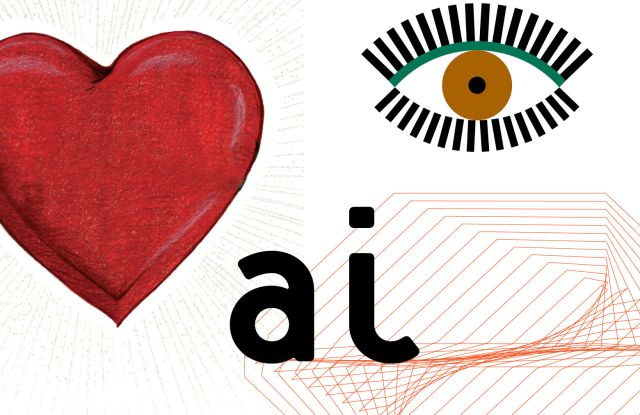
MUHAI Visual Identity
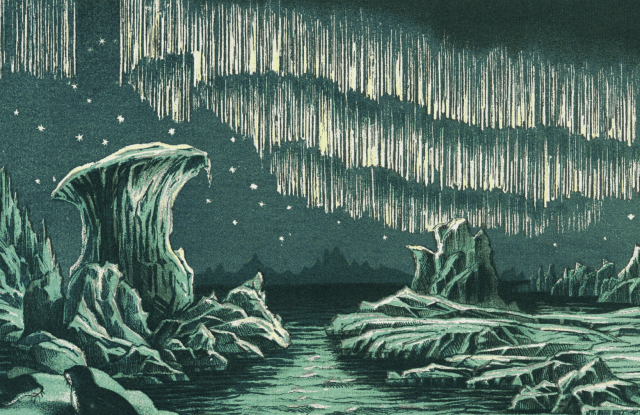