Webinar: The K in 'neuro-symbolic' stands for 'knowledge'
MUHAI partner Frank van Harmelen, Professor in Knowledge Representation & Reasoning at Vrije Universiteit Amsterdam, gives a presentation “The K in 'neuro-symbolic' stands for 'knowledge'”.
The video is available for free on youtube and is the first webinar in the NAI Webinar Series moderated by Pascal Hitzler, Editor-in-Chief of the Neurosymbolic Artificial Intelligence journal.
Tutorial 1: Recipe Execution Benchmark
This benchmark for recipe understanding in autonomous agents aims to support progressing the domain of natural language understanding by providing a setting in which performance can be measured on the everyday human activity of cooking.
Discover how it works thanks to this video tutorial!
Tutorial 2: Muhai general tutorial - EKAW2024
This tutorial was hosted at EKAW 2024, participants learnt how to build human-centric AI agents using the MUHAI approach, through a mix of showcases related to understanding everyday activities in the real world and hands-on exercises to practise with the solutions developed within the project. Focusing on the hybrid integration of symbolic AI (including e.g. ontological modelling of narratives, automated knowledge extraction from large-scale knowledge graphs, storage of episodic and semantic memories) and subsymbolic AI methods (language understanding, speech processing, reinforced learning, active learning, etc.).
MUHAI Hypotheses in a Nutshell
The MUHAI approach relies on 3 hypotheses:
1. Modularity Hypothesis: The process of understanding consists in constructing a coherent narrative about a situation that integrates and is compatible with a multitude of modular knowledge sources. These knowledge sources range from visual and motor experiences rendered through quantitative and qualitative simulation, to language processing, to semantic and episodic memory stored and accessed via knowledge graphs.
2. Multiplicity Hypothesis: The neural, machine learning approach to AI, as explored in behaviour-based cognitive robotics or generative AI, appears to be an effective operational model for the (system 1, data-driven, fast) cognitive mind whereas the knowledge-driven methods used in expert decision systems or semantic web applications is a more effective model for the (system 2, knowledge-driven, deliberative, slow) sapient mind. Human-centeredness when dealing with everyday activities can be reached using a hybrid approach that tightly integrates cognition, and sapience together with embodiment (perception), consciousness and sentience.
3. Constructivist Learning Hypothesis: knowledge in the form of categories and theories are not derived from data by generalisation but only exists within the human mind. Learners then constantly try to develop their own individual mental model of the real world from their perceptions of that world. Learners continually update their own mental models based on new incoming information (active learning), on social interaction (multi-agent learning) and on the goal of their tasks (goal-directed learning).
Discover the Muhai approach thanks to this slideshow from the original presentation!
Tutorial 3: MIRA KG
Discover how to use the MIRA demo developed within MUHAI. A tutorial offered to you by Lise Stork (VUA).
Women in science 2025 poster
For the Women in Science event at Université de Namur, Katrien Beuls and Paul Van Eecke presented this study about learning from situated interactions. 13.02.25 - https://wgis.unamur.be/
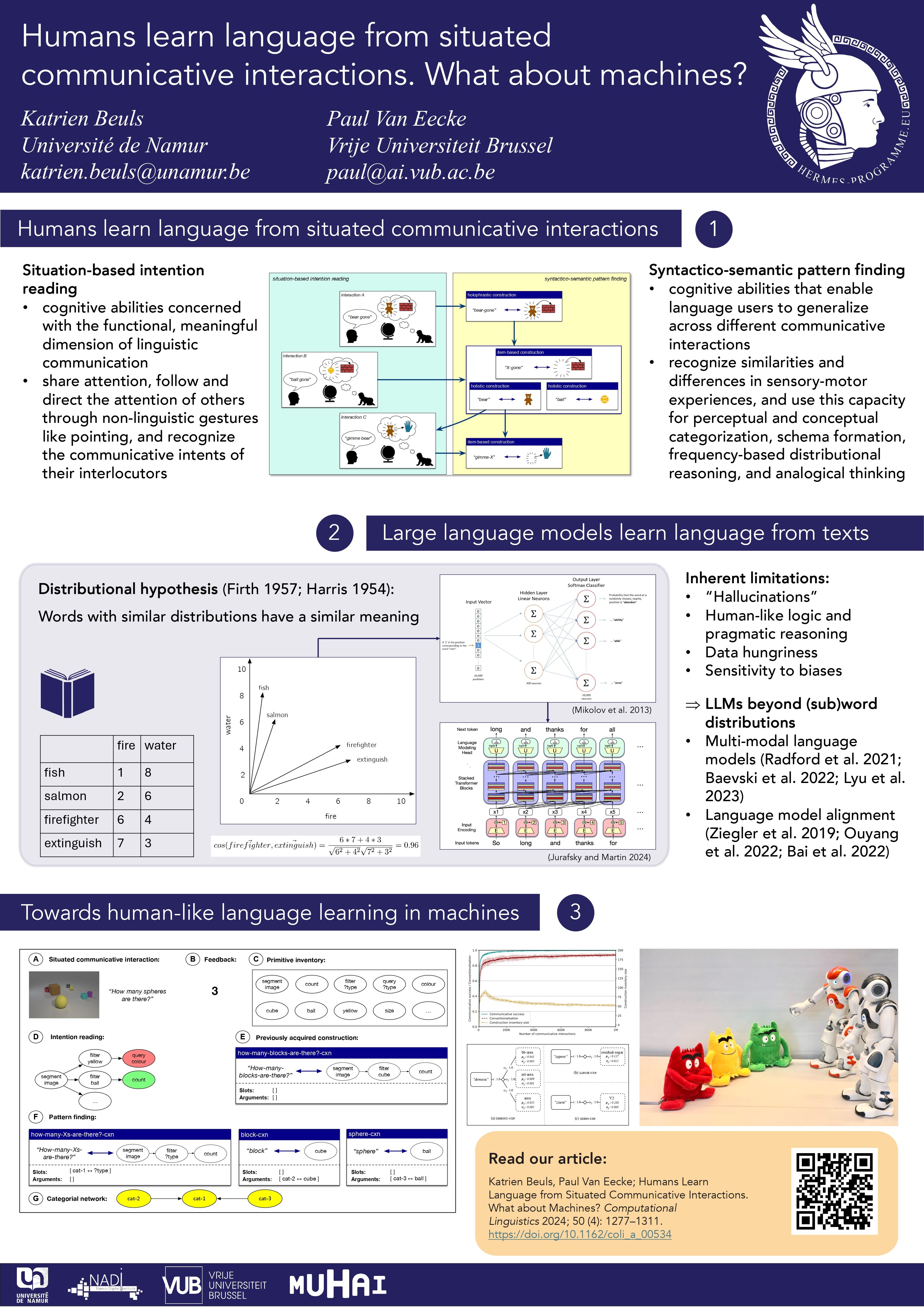