Linguistic Alignment for Chatbots
Laura Spillner, UHB.
Over the last decade, conversational agents have slowly but surely become very common in our daily lives. Conversational agents - these are computer agents, robots or software which can understand and talk to a user in natural language - include assistants on our smartphones like Siri or Google Assistant, voice-controlled smart home devices like Google Home and Amazon Alexa, as well as online chatbots like the ones frequently used in customer service. Clearly, natural language plays an important role when it comes to fluent and easy interaction with computers and mobile devices. But in spite of their popularity and the considerable amount of recent research in AI-based understanding and generation of natural language, these interfaces still face some difficult challenges.
Human communication comes with a number of peculiarities: conversations are often informal and use non-standard language compared to formal written texts; ignoring grammar and spelling rules. And even when writing chat messages, many people employ the same kind of informal language as they do when talking in person. New types of expressions such as emojis and their often variable meaning can also hinder successful human-computer communication.
Many researchers have shown that users tend to react socially to computer agents, meaning that they interact with computers or robots in the same way as they do with other humans [1]. This is the case especially if these agents also employ natural language, or if they imitate humans' social and affective cues [2, 3]. But when it comes to conversational agents, this can easily lead to misunderstandings or communication breakdowns. As many users now, even popular chatbots and voice assistants still fail quite often in real-world usage: They misunderstand what we're trying to ask or what we want it to do, they miss important context, and their answers are often robotic and unnatural.
In the field of psycho-linguistics, researchers have studied how two people engaged in a conversation are able to achieve mutual understanding and prevent communicative failures. The social and collaborative nature of human conversation is well established, in theories such as Communication accommodation theory [4] and Interactive alignment theory [5]. Based on these theories, successful communication between humans depends on both participants’ ability to adapt to the language of their conversation partner. This means that two speakers imitate each other’s use of language in many ways, without being aware of that they are doing it. They repeat each others' word choices, phrasings, and sentence structure, and slowly become more similar to one another in their overall language style. In this way, they are also able to achieve a more similar understanding of the situation under discussion. Thus, imitating one's conversation partner effectively paves the way for successful communication.
In human interaction, this kind of "linguistic alignment" is what makes successful communication possible. Aside from language itself, it has also been shown that people imitate others when they interact with them, mirroring their facial expressions and gestures. The same effect happens when people imitate each other’s language style in conversation. This adaptation or imitation directly impacts how positively someone is perceived by others: If someone aligns more strongly to their conversation partner, their partner will think better of them. Moreover, alignment is not just important in terms of perception, but also directly impacts how well people are able to work together. For example, when it comes to solving tasks together with others, people who's language aligns more closely to each other are more successful in their tasks [6]. They also report lower workload and higher engagement during the task, when they align better with their partner [7].
Of course, our interaction with chatbots and other conversational agents is very much impacted by how we chat with other people. Chat apps are among the most popular mobile applications and the most popular types of social media; and chat conversations have evolved their own kind of language too. This kind of language is less adherent to classic dialog structure and formal grammar or spelling rules. All of this makes natural conversations difficult for AI. Therefore, we reasoned that considering the psychological model of human communication will be essential in order to improve interaction with conversational agents. If users interact with chatbots as if they were social agents, in the same way in which they interact with other people, then they might subconsciously expect them to adhere to the same communication patterns as other people would.
Based on this idea, we developed a new chatbot called KONRAD. Konrad is able to produce a linguistic alignment effect, similar to the alignment that happens in human conversations. In order to do this, the chatbot repeats the user’s word choices and uses similar similar sentence structures as the user. Konrad works as a simple goal-oriented chatbot, which intends to help users decide which movie to watch at a local cinema. Conversations with Konrad are user-initiative and based on the intent action structure: This means that there is no chit-chat or small talk conversation as there is with other AI chatbots like Cleverbot. Instead users have the initiative and ask the chatbot questions, similar to Siri and other smart assistants. In this case, users can ask about which movies are currently running, information about those movies, and general information about the cinema. The chatbot then predicts the intent of the user’s question (one of a number of pre-defined categories, such as the starting time of a specific movie). It looks up the information needed to answer the question, and then formulates an answer. Using Konrad, we conducted an online study to investigate how users would perceive and evaluate a chatbot that mimics human behavior through linguistic alignment.
We expected that, based on the theory that people treat conversational agents and robots as social agents, the study participants would align their language more strongly to the chatbot if it also exhibited an alignment effect. Moreover, solving tasks and acquiring information are key use cases of chatbots and other conversational agents; and previous studies have shown that alignment reduces workload when solving tasks together. Thus, we also hypothesized that the participant’s workload when solving a task with the help of the chatbot should be lower if the chatbot aligns to them linguistically.
We built three different versions of Konrad: The first version does not have any alignment, but instead works in the same way as most conversational agents and chatbots currently do; by using pre-defined templates for answers. This is comparable to other popular conversational agents such as Siri, as well as state-of-the-art chatbot frameworks like Google’s Dialogflow. They generally build their answers to user requests in pre-defined ways, inserting information into a template answer.
The other two versions of our chatbot Konrad implement two different variants of the desired alignment effect. The first one only implements a basic alignment effect, in which the chatbot adapts to the user's choice of words: Normally, the chatbot uses pre-defined answers. But when the chatbot recognizes that the user prefers a specific term for something, a word that is a synonym to the one the chatbot would otherwise use, then it replaces its pre-defined term with that synonym. In this way, the answers are still similar to the pre-defined template ones, only with some of the words replaced. The second alignment version does away with the template answers all-together. In this version, we aimed at producing a structural alignment effect, i.e. imitating the syntactic structure of the user’s question in the chatbot’s answer. In order to achieve this, the chatbot dynamically generates its answer using the structure of the question as a basis - it rearranges the question into an answer format, and then inserts the information necessary to answer the question at the appropriate position. For example, if someone asks Konrad “Which actor is Luke Skywalker played by?” it would answer “Luke Skywalker is played by Mark Hamill”. But if you ask it “Who portrays Luke Skywalker in Star Wars?” it answers “Mark Hamill portrays Luke Skywalker in Star Wars”. This way, it keeps the syntactic elements from the question (here, the passive vs. active voice) and also automatically reuses the terms which the user prefers (here, "plays" vs "portrays") without needing to identify possible synonyms.
In our study, the chatbot Konrad was tested by 75 participants. They could access it online, either on their computer or on a smartphone. Each participant was randomly assigned one of the three versions of the chatbot, and only interacted with this one version. They were tasked with using the chatbot to find a movie that they would want to see. During the conversation, they could ask the chatbot about when which movie was running, ask it for details about the different movies, or to give them reviews and recommendations. Once they had decided on a movie, they were asked to fill in a short online survey: with this survey, we assessed their general opinion of the chatbot, and also measured their perceived workload and engagement during the conversation. Afterwards, we evaluated both the information from the survey, as well as the conversations itself. By analyzing the conversations that users had with the chatbot, we were able to measure how strongly either of them aligned to the other one.
Our study supported our hypothesis that linguistic alignment would impact the interaction with the chatbot. Firstly, we found that user alignment was correlated with chatbot alignment, showing that users did in fact react to the alignment effect displayed by Konrad by aligning more strongly to it in turn. Secondly, the users' workload was lower and engagement was higher when they tested the variants of the chatbot with added alignment. In fact, workload was even negatively correlated with alignment, meaning that those which had higher alignment throughout the conversation experienced lower workload in solving the task at hand. The same has been shown in previous work for human conversation [7] - our results show that this connection between alignment and task workload applies to human-computer-communication as well.
In this study, we tested Konrad in the film domain, because many people are familiar with this domain and there is not a huge influence of prior knowledge. However, alignment might differ depending on the domain as well as the user's motivation. For example, the effect of alignment might be different when searching for information on an unfamiliar topic: you might not want the chatbot to imitate your choice of words, if you are not certain which terms are correct. Moreover, we wonder whether there is a difference between solving a task with the chatbot, compared to engaging in small talk. Finally, the length of the sentences and the conversation might have an influence on the alignment, which we did not investigate here. These topics we plan to look into in future studies. We expect that additional strategies and implementations of alignment will be required in order to extend this idea to a more general approach - for Konrad, we relied on a combination of neural networks (in order to understand the topic of the user's question) and grammatical rules (in order to construct the chatbot's answers). In future implementations however, other usage domains and more general conversations will likely require more flexible approaches.
The results of this study showed that the phenomenon of linguistic alignment has an impact on conversations between humans and computers. Thus, it should be taken into account when developing natural language-based interfaces such as chatbots. Alignment plays an important role in achieving successful communication between humans, and implementing it in conversational agents can clearly have real-world benefits for user interaction.
References
[1] Clifford Nass and Youngme Moon. 2000. Machines and mindlessness: Social responses to computers. Journal of social issues 56, 1 (2000), 81–103.
[2] Theo Araujo. 2018. Living up to the chatbot hype: The influence of anthropomorphic design cues and communicative agency framing on conver- sational agent and company perceptions. Computers in Human Behavior 85 (2018), 183–189.
[3] Eun Go and S Shyam Sundar. 2019. Humanizing chatbots: The effects of visual, identity and conversational cues on humanness perceptions. Computers in Human Behavior 97 (2019), 304–316.
[4] Howard Giles and Peter Powesland. 1997. Accommodation theory. In Sociolinguistics. Springer, 232–239.
[5] Martin J Pickering and Simon Garrod. 2004. Toward a mechanistic psychology of dialogue. Behavioral and brain sciences 27, 2 (2004), 169–190.
[6] David Reitter and Johanna D. Moore. 2014. Alignment and task success in spoken dialogue. Journal of Memory and Language 76 (2014), 29–46.
[7] Paul Thomas, Mary Czerwinski, Daniel McDuff, Nick Craswell, and Glo- ria Mark. 2018. Style and alignment in information-seeking conversation. In Proceedings of the 2018 Conference on Human Information Interaction and Re- trieval. 42–51.
This article contains excerpts and figures from a previously published paper: Laura Spillner and Nina Wenig. 2021. Talk to Me on My Level – Linguistic Alignment for Chatbots. In Proceedings of the 23rd international conference on mobile human-computer interaction. New York, USA: Association for Comput- ing Machinery.
Credits
Intro photo by Pavel Danilyuk from Pexels
More Articles
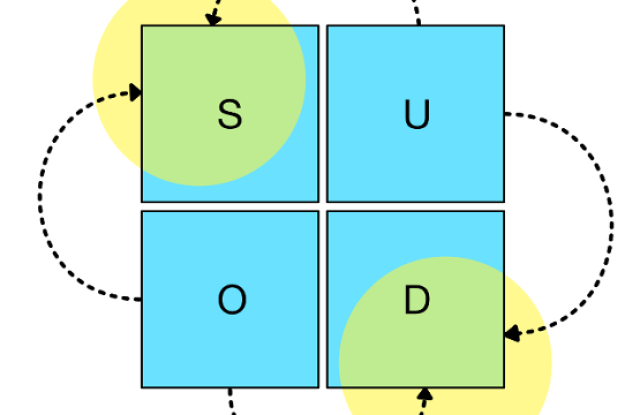
Uncommon Ground
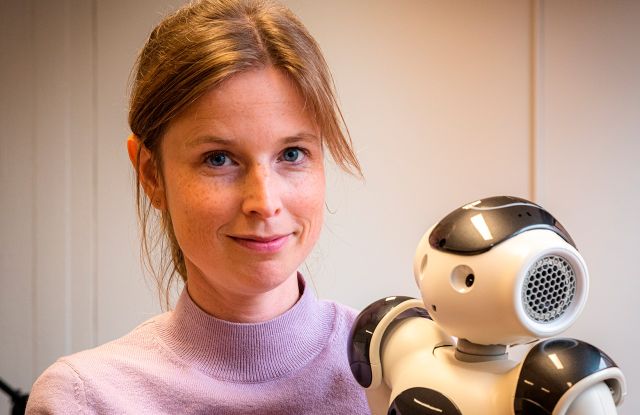
Do you speak AI?
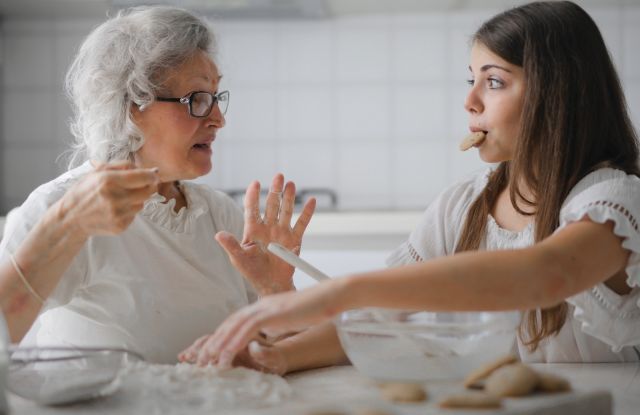
Pragmatics: the secret ingredient
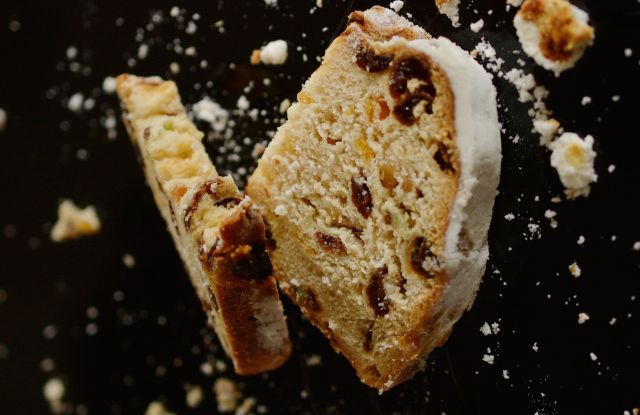
Deconstructing Recipes
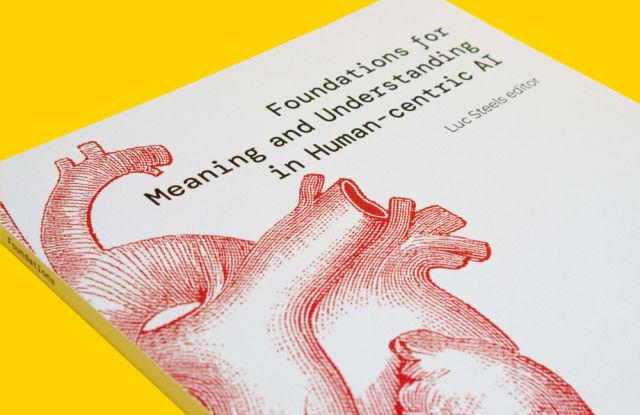
Foundations for Meaning and Understanding in Human-centric AI
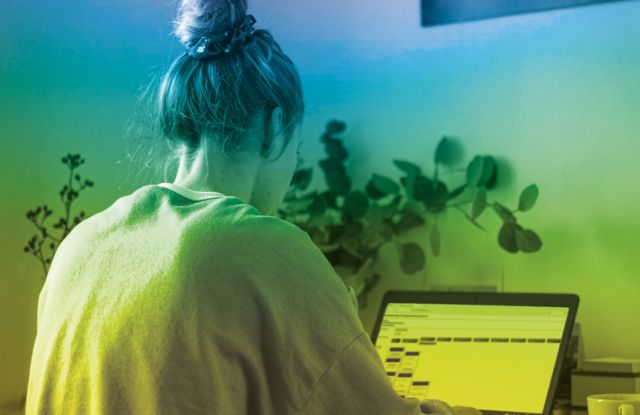
The FCG Editor: a new milestone for linguistics and human-centric AI
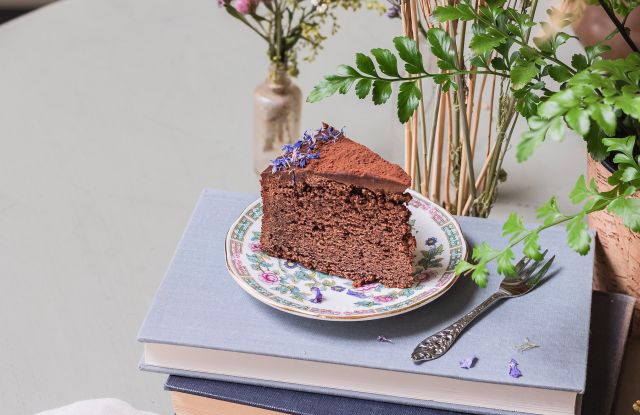
Framing reality
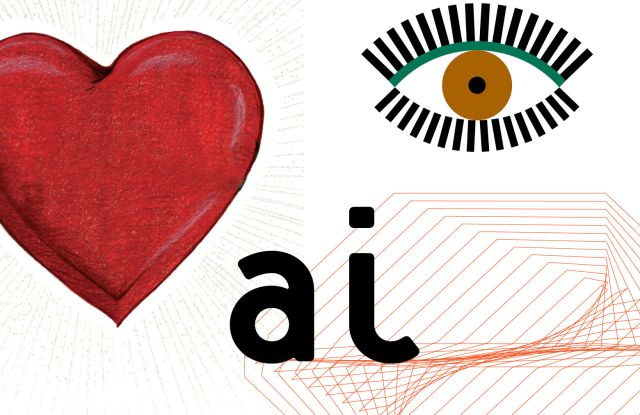
MUHAI Visual Identity
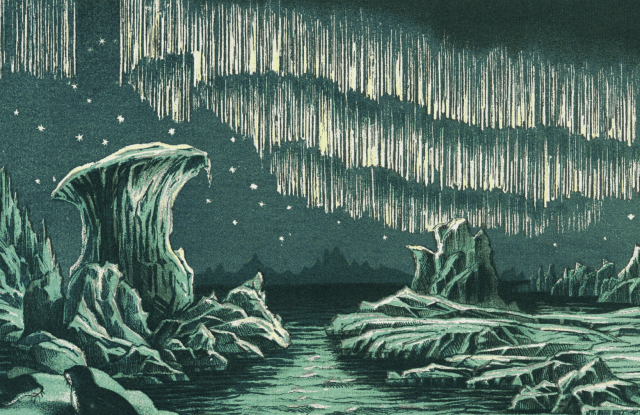